Parsing is All You Need for Accurate Gait Recognition in the Wild
ACM International Conference on Multimedia (ACM MM) 2023
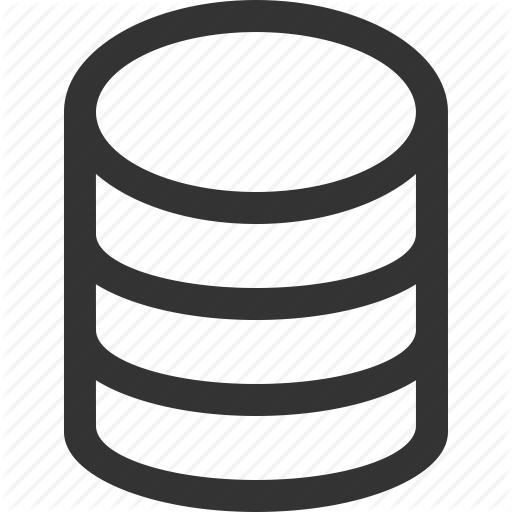
DataSet
|
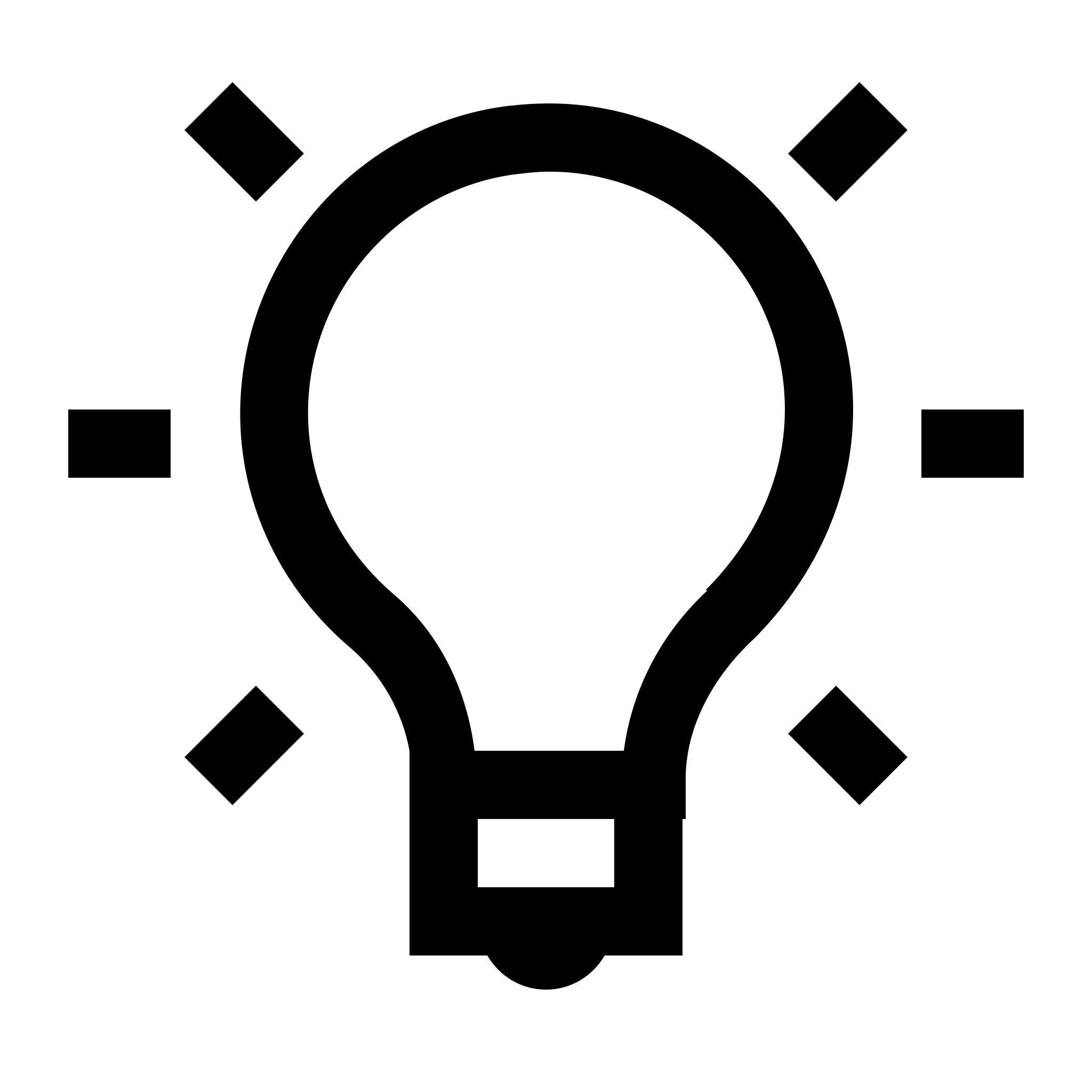
Method
|
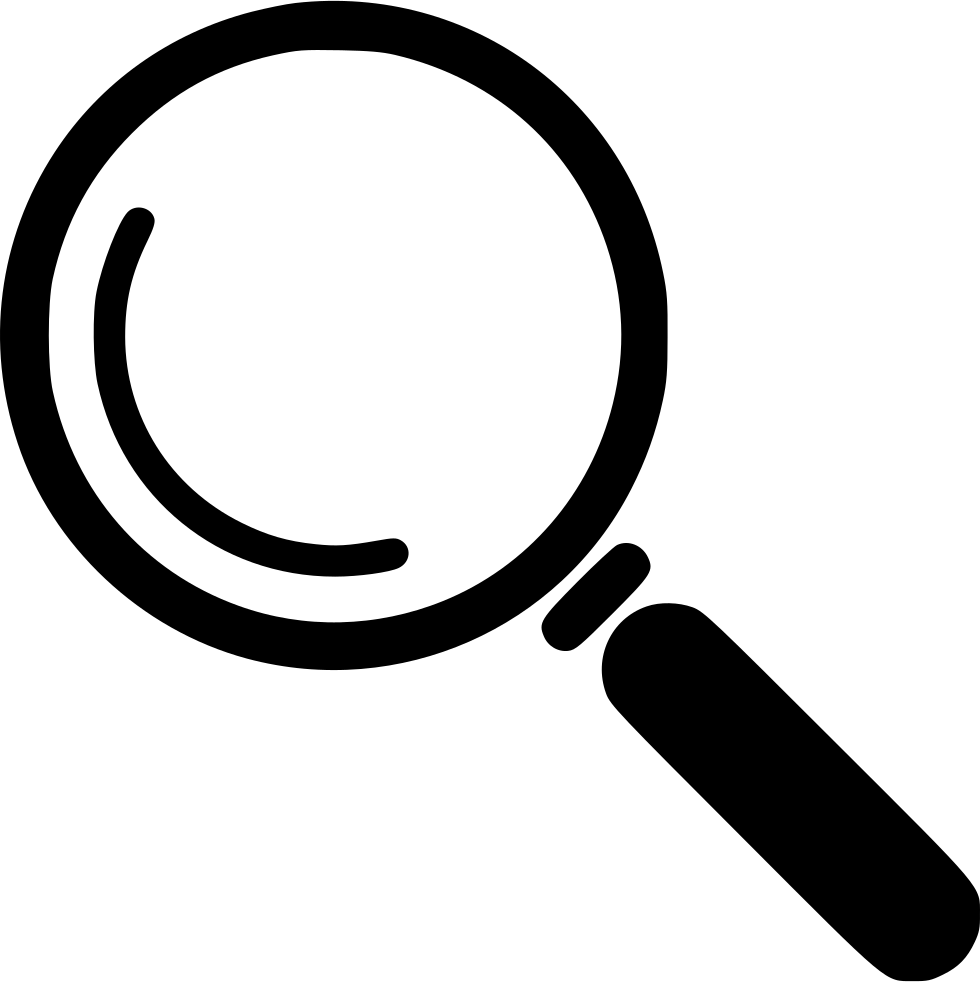
Analysis
|
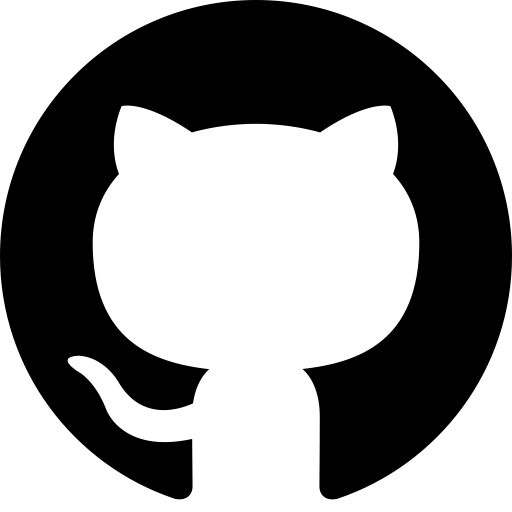
GitHub Repo
|
Binary silhouettes and keypoint-based skeletons have dominated
human gait recognition studies for decades since they are easy to
extract from video frames. Despite their success in gait recognition
for in-the-lab environments, they usually fail in real-world scenarios
due to their low information entropy for gait representations.
To achieve accurate gait recognition in the wild, this paper presents
a novel gait representation, named Gait Parsing Sequence (GPS).
GPSs are sequences of fine-grained human segmentation, i.e., human parsing,
extracted from video frames, so they have much higher
information entropy to encode the shapes and dynamics of fine-grained human parts
during walking. Moreover, to effectively explore the capability of the GPS representation,
we propose a novel human parsing-based gait recognition framework, named ParsingGait.
ParsingGait contains a Convolutional Neural Network (CNN)-based backbone and two light-weighted heads.
The first head extracts global semantic features from GPSs, while the other
one learns mutual information of part-level features through Graph
Convolutional Networks to model the detailed dynamics of human walking.
Furthermore, due to the lack of suitable datasets, we build the first parsing-based dataset for gait recognition in the wild,
named Gait3D-Parsing, by extending the large-scale and challenging Gait3D dataset.
Based on Gait3D-Parsing, we comprehensively evaluate our method and existing gait recognition methods.
Specifically, ParsingGait achieves a 17.5% Rank-1 increase compared with the state-of-the-art silhouette-based method.
In addition, by replacing silhouettes with GPSs, current gait recognition methods achieve about 12.5% ∼ 19.2% improvements
in Rank-1 accuracy. The experimental results show a significant improvement in accuracy brought
by the GPS representation and the superiority of ParsingGait.
Gait3D-Parsing Dataset
(1) Examples of gait representations in the Gait3D-Parsing dataset.
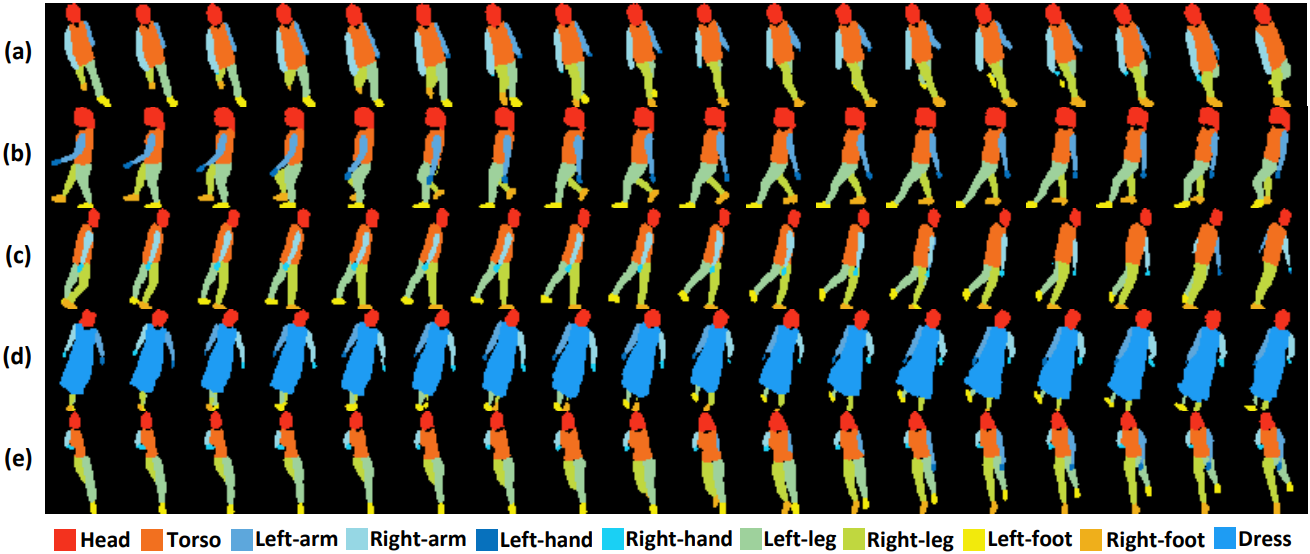
|
(3) Download Gait3D-Parsing.
All users can obtain and use Gait3D-Parsing dataset only after signing the
Agreement
and sending it to the official contact email address (gait3d.dataset@gmail.com).
To obtain the original Gait3D dataset, please access
the website of Gait3D.
|
The Architecture of ParsingGait
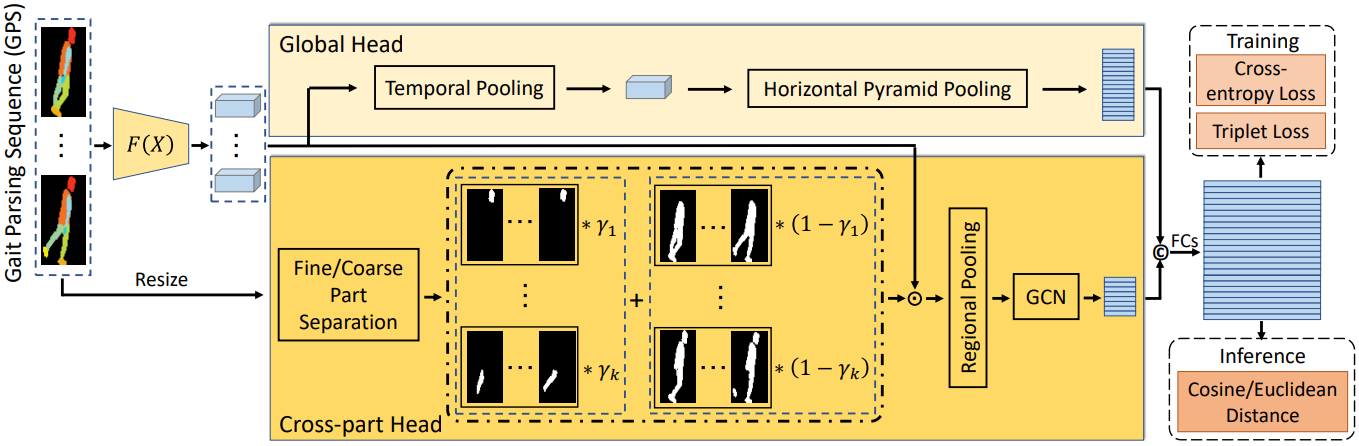
|
The architecture of the ParsingGait framework for gait recognition in the wild.
|
Evaluation and Visualization
(1) Comparison of the state-of-the-art gait recognition methods.
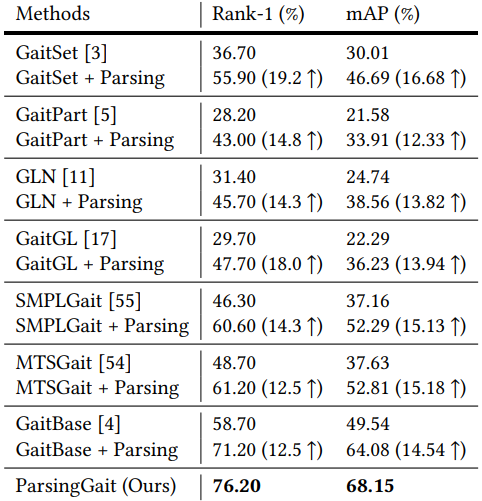
|
Comparison of the state-of-the-art gait recognition methods.
With parsing, the performance of all methods was greatly improved (12.5% ~ 19.2%).
In addition, our ParsingGait achieved the best performance.
|
(2) Exemplar results.
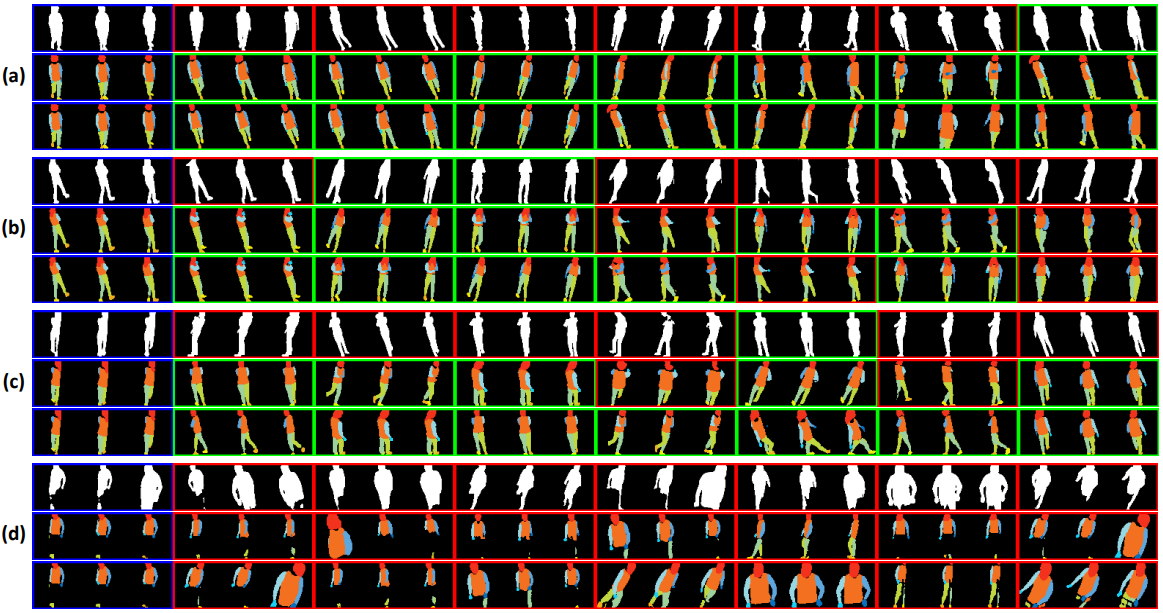
|
Some exemplar results of GaitBase, GaitBase+Parsing, and our ParsingGait. For convenience, we choose the middle
frame and the frames with four intervals before and after it for visualization. The blue bounding boxes are queries. The green
bounding boxes are the correctly matched results, while the red bounding boxes are the wrong results. The (a) - (d) represent
the results under different queries, where the first row of each is the search result of GaitBase, the second row is the result of
GaitBase+Parsing, and the third row is the result of ParsingGait. (Best viewed in color.)
|
Paper
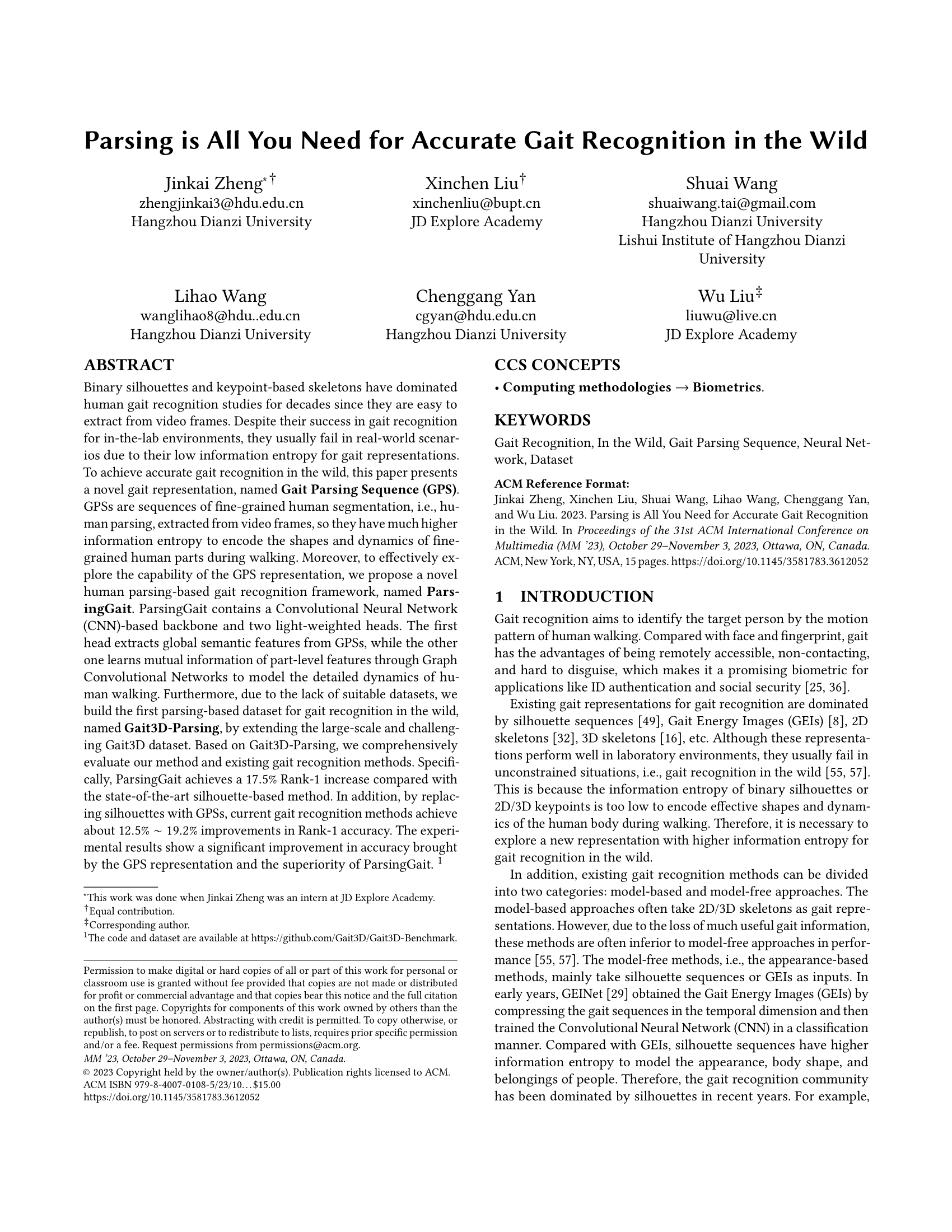 |
Zheng, Liu, Wang, Wang, Yan, Liu.
Parsing is All You Need for Accurate Gait Recognition in the Wild
In ACM MM, 2023.
(arXiv)
|
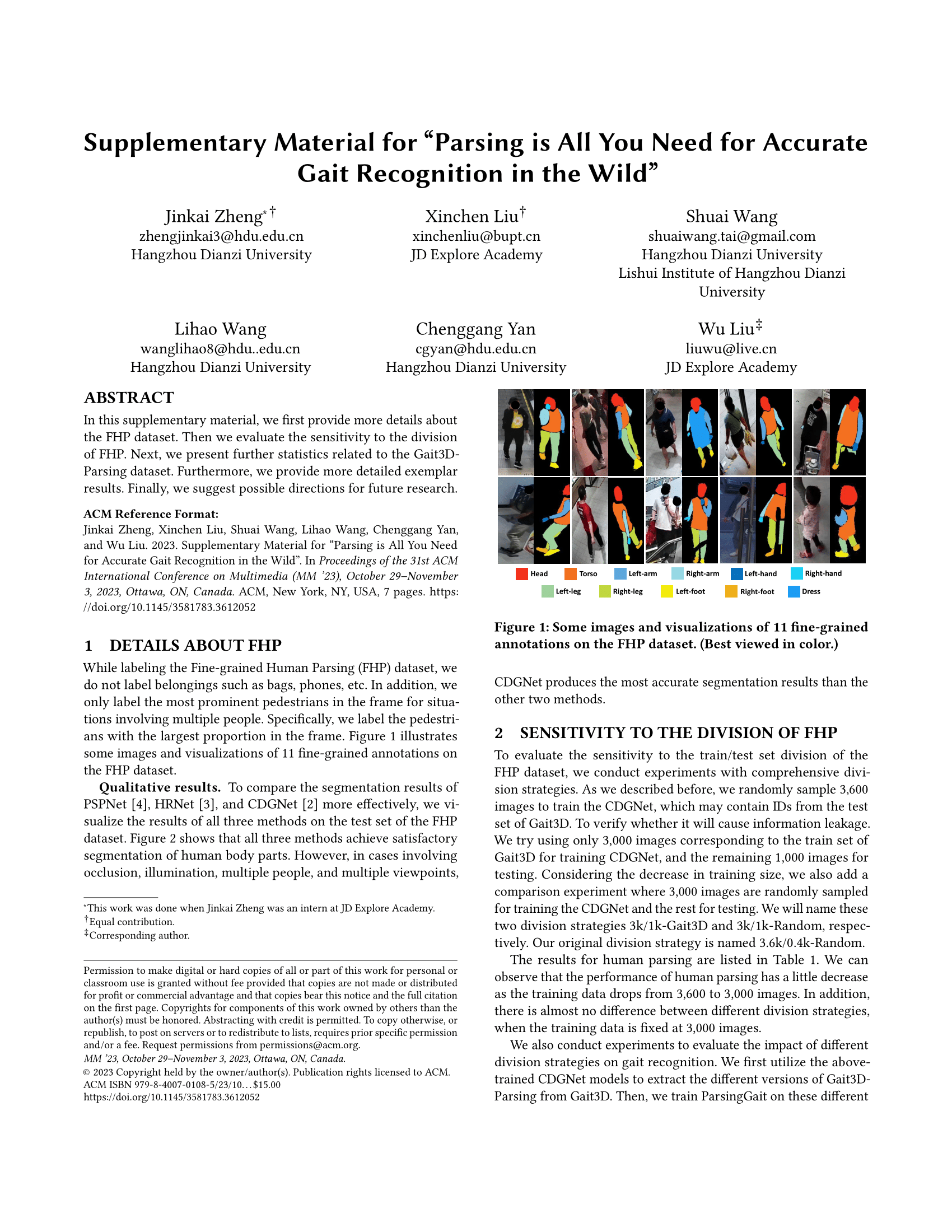 |
(Supplementary)
|
Cite
@inproceedings{zheng2023gait3dparsing,
title={Parsing is All You Need for Accurate Gait Recognition in the Wild},
author={Jinkai Zheng, Xinchen Liu, Shuai Wang, Lihao Wang, Chenggang Yan, Wu Liu},
booktitle={ACM International Conference on Multimedia (ACM MM)},
year={2023}
}
Acknowledgements
This work was supported by the National Key Research and Development Program of China under Grant (2020YFB1406604), Beijing
Nova Program (20220484063), National Nature Science Foundation of China (61931008, U21B2024), "Pioneer", Zhejiang Provincial
Natural Science Foundation of China (LDT23F01011F01).
This work was done when Jinkai Zheng was an intern at JD Explore Academy.
|